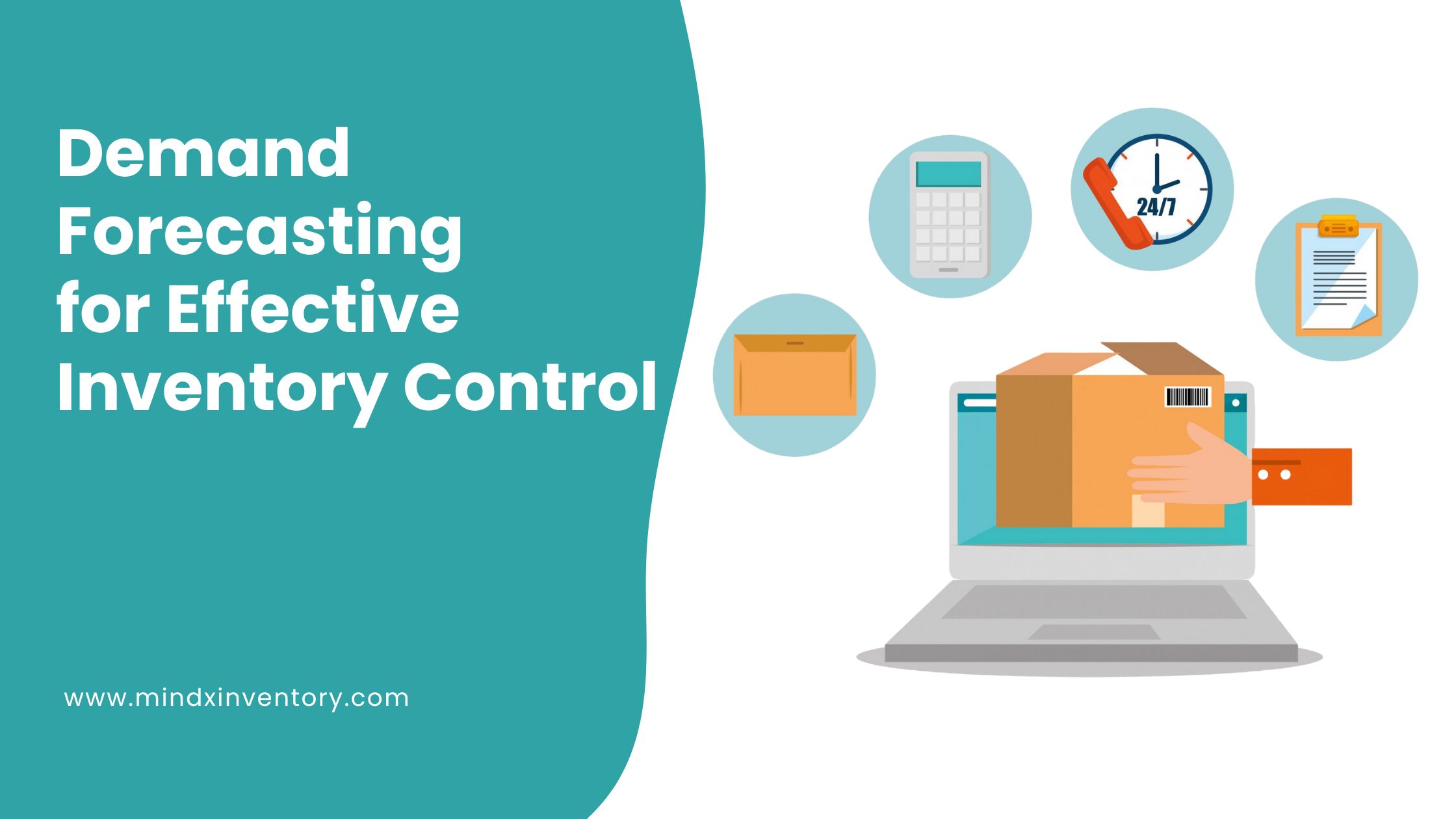
The Role of Inventory Management in Modern Business
Inventory management plays a critical role in today’s business environment, where speed, flexibility, and customer satisfaction are essential. Companies must strike a balance in managing their stock to meet demand, reduce operational costs, and enhance overall efficiency.
Efficient inventory management affects both product availability and the financial health of the company. Holding too much stock leads to high carrying costs such as storage, insurance, and the risk of obsolescence. On the other hand, insufficient stock can result in lost sales, customer dissatisfaction, and damaged brand reputation.
Modern businesses rely on advanced technologies such as Enterprise Resource Planning (ERP) systems, cloud-based solutions, and automation for real-time inventory tracking. These tools provide better visibility into stock movements, help reduce errors, improve replenishment cycles, and enhance forecasting accuracy. Predictive analytics are now widely used to forecast demand, optimize storage, and adjust inventory levels in real-time, keeping businesses competitive.
Harnessing Demand Forecasting for Smarter Inventory Control
Demand forecasting is integral to inventory management, helping businesses predict customer needs and adjust stock levels accordingly. Accurate forecasting reduces the risks of stockouts and overstocking, which can negatively impact profitability and customer satisfaction.
Forecasting relies on historical sales data, market trends, and external factors like seasonality and economic shifts. Simple statistical methods and more advanced machine learning models help analyze data and detect patterns in consumer behavior. Time series analysis is a common technique where past sales data predicts future demand. However, external disruptions (like changes in consumer behavior or supply chain issues) may affect predictions.
Machine learning and AI are becoming central to demand forecasting, allowing for more precise predictions. These models continuously adapt to new data, providing businesses with better accuracy and enabling automated inventory replenishment. Collaborative forecasting—where businesses share sales data with suppliers—also enhances forecast accuracy, aligning supply chains more effectively.
Mastering Timing and Quantity in Inventory Management
Balancing the right timing and quantity of inventory is essential to meeting customer demand without overstocking or understocking.
- Reorder Points and Safety Stock Levels: Reorder points (ROP) determine when to replenish stock. Safety stock accounts for uncertainty in demand or supply. Both metrics are calculated by analyzing usage rates and procurement lead times, helping businesses avoid stockouts while minimizing excess inventory.
- Economic Order Quantity (EOQ): EOQ calculates the optimal order quantity that minimizes total costs, including ordering and holding costs. This model helps businesses strike a balance between ordering frequently and overstocking.
- Inventory Turnover Rate: This metric measures how quickly inventory is sold and replaced. High turnover indicates effective management, while low turnover suggests overstocking or slow-moving products.
By monitoring these key factors, businesses can adjust their inventory levels, improving operational efficiency and reducing costs.
Strategies to Improve Inventory Management with Forecasting
Effective inventory management relies on integrating forecasting into operational processes. Below are strategies to optimize inventory and forecasting:
- System Integration: Integrating forecasting tools with inventory management systems provides real-time data, reducing manual errors and improving stock adjustments. Automation helps eliminate discrepancies between demand and supply.
- Model Refinement: Continuously refine forecasting models by analyzing sales data and market conditions. Accurate forecasting improves with regular updates, helping businesses plan better.
- Advanced Forecasting Techniques: Using machine learning and econometric models improves forecast accuracy by analyzing external and internal factors affecting demand. These techniques enhance prediction reliability.
- Collaborative Forecasting: Collaborating with suppliers and customers provides better alignment in inventory management. Sharing data ensures timely deliveries and reduces the risk of stockouts or overstocking.
By implementing these strategies, businesses can streamline inventory management, reduce costs, and improve customer satisfaction.
Overcoming Challenges in Demand Forecasting
While demand forecasting offers numerous benefits, businesses face several challenges:
- Data Accuracy: Forecasting relies on accurate data. Incomplete or inaccurate data can lead to poor predictions. Businesses must invest in robust data collection and validation processes to ensure the quality of data used in forecasting models.
- Market Dynamics: Rapid shifts in consumer preferences or external events, such as economic downturns or new product launches, make predicting demand challenging. Businesses need to stay flexible and adjust their strategies as market conditions change.
- Model Complexity: Advanced forecasting models, especially those involving AI and machine learning, can be complex and require specialized knowledge. Businesses may need to collaborate with professionals or use third-party solutions for better forecasting accuracy.
- Uncertainty: External disruptions—like supply chain issues, natural disasters, or geopolitical shifts—introduce uncertainty. Flexibility and contingency planning are essential to mitigate the impact of unforeseen events on demand forecasts.
Addressing these challenges requires careful data management, flexibility in forecasting models, and a proactive approach to market changes.
Emerging Trends in Inventory and Forecasting
Several emerging trends are shaping the future of inventory management and demand forecasting:
- Artificial Intelligence (AI) and Machine Learning: These technologies analyze vast datasets to uncover demand patterns and make accurate predictions. Machine learning models continuously adapt, improving forecasting accuracy over time.
- Real-Time Analytics: Real-time data analytics allows businesses to respond swiftly to changes in demand, disruptions, or market shifts, enhancing their ability to stay competitive.
- Supply Chain Integration: Integrating inventory management with procurement, production, and logistics improves efficiency by providing end-to-end visibility and streamlining operations.
- Sustainability: Sustainable practices are becoming more important, with businesses seeking ways to reduce waste, optimize resource use, and minimize overproduction.
By adopting these trends, businesses can enhance their forecasting capabilities, improve inventory efficiency, and better navigate a rapidly changing marketplace.
The Link Between Forecasting and Inventory Success
Demand forecasting and inventory management are interdependent. Accurate forecasting provides the insights needed for effective inventory control, while efficient inventory management ensures that forecasts are executed effectively.
Forecasting helps businesses understand demand patterns, allowing for better purchasing decisions, production planning, and space optimization. It prevents overproduction or understocking, both of which can incur significant costs. Effective inventory management, meanwhile, ensures that businesses are prepared for changes in demand, keeping stock levels aligned with forecasts.
When forecasting and inventory management work together, businesses can reduce stockouts, improve cash flow, and increase customer satisfaction, making the business more dynamic and responsive in a fast-paced market.
Why Demand Forecasting Drives Business Growth
Demand forecasting is more than a tool for inventory management—it’s a key factor in driving business growth. By maintaining optimal inventory levels, companies can reduce costs associated with stockouts and overstocking, freeing up capital while ensuring products are available for customers.
Accurate forecasting allows businesses to remain agile, adapting quickly to market shifts or changing consumer preferences. It enables businesses to optimize supply chain operations, reduce lead times, and ensure timely deliveries, all of which contribute to long-term success and growth.
Transforming Inventory Management with MindX Inventory
MindX Inventory combines advanced demand forecasting with intuitive inventory management. With real-time data analytics, automation, and machine learning, businesses can improve decision-making, optimize inventory levels, and reduce operational costs. MindX’s automated processes and advanced forecasting tools help businesses stay ahead in a rapidly evolving market.
Key Insights for Applying Forecasting to Inventory Strategy
To maximize the benefits of forecasting, businesses must integrate forecasting into their overall inventory strategy. Key actions include:
- Integrate Systems: Ensure seamless data flow between forecasting and inventory systems for better accuracy and decision-making.
- Leverage Advanced Techniques: Use AI and machine learning for deeper insights into demand trends.
- Collaborate Across the Supply Chain: Share data with suppliers and customers to align inventory management with actual demand.
- Monitor and Refine Models: Continuously improve forecasting models based on real-world data.
By applying these insights, businesses can optimize inventory management, reduce costs, and ensure consistent customer satisfaction.
Ready to transform your inventory management? Book a Demo